Mathematics at the service of Artificial Intelligence
Making "black box" models understandable
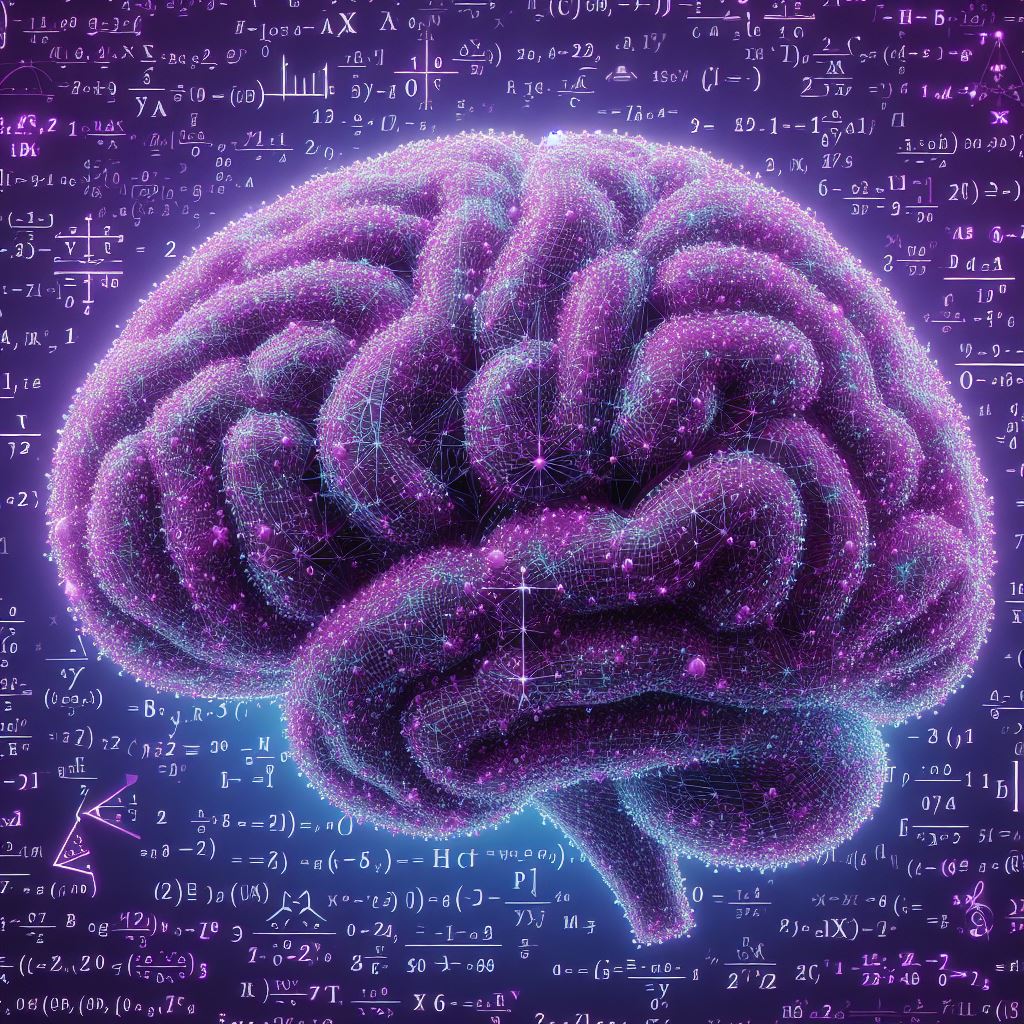
In the digital era, Artificial Intelligence (AI) plays an increasingly central role in our daily lives. However, many of its internal mechanisms remain shrouded in mystery, encapsulated in what experts refer to as "black box" models. These complex systems, while extremely powerful, often lack something fundamental: interpretability.
Recent studies are paving the way to make these models not only more effective but also more understandable. Through the use of mathematical optimization methods, researchers are developing techniques to "open up" these black boxes, making AI more transparent and accessible to everyone.
Mathematical optimization, a branch of applied mathematics that deals with finding the best possible outcome within a given set of parameters, is proving to be crucial in this process. By employing advanced algorithms, it is possible to analyze and modify AI models to make them not only more efficient but also easier to interpret.
Mathematical optimization plays a crucial role, allowing to transition from an opaque and complex machine learning model to one that is more interpretable and transparent.
A recent study, titled "Supervised feature compression based on counterfactual analysis" (Piccialli, Veronica, Dolores Romero Morales, 2023), marks a significant step in this direction. The main goal of this work is to improve transparency and understandability using a technique called "counterfactual analysis." Counterfactual explanations seek to answer questions such as: "What would need to change in a given input to achieve a different outcome?" For example, if a model determines that a customer is not eligible for a loan, counterfactual analysis could indicate what changes in the customer's data could lead to a positive decision.
The article proposes a method to utilize the information obtained from counterfactual explanations to construct a decision tree, which is a type of machine learning model much easier to understand because it can be visualized as a series of binary questions and answers (yes/no). The created tree seeks to mimic the behavior of the original model but in a much more transparent form. The significant advantage of this approach is that it makes machine learning models more transparent and trustworthy, especially in sensitive sectors such as healthcare and finance, where understanding the "why" behind a decision can be crucial.
Mathematical optimization plays a crucial role in the article, being the key to implementing and improving the proposed methodology of supervised feature compression based on counterfactual analysis, allowing the authors to transition from an opaque and complex machine learning model to one that is more interpretable and transparent.
Numerical results on real-world datasets confirm the effectiveness of this approach, not only in terms of accuracy but also in reducing the complexity of the model. This study opens up new perspectives for making AI more transparent and trustworthy, a crucial step towards the ethical and responsible adoption of these technologies in our daily lives.
Deix stands out in the technological landscape thanks to its unique ability to integrate advanced expertise in applied mathematics, optimization, and numerical simulation with the most modern techniques of artificial intelligence and machine learning. This synergy creates a powerful combination that we call "Mathematical Intelligence." This approach not only improves the understanding and ethics of AI models but also significantly increases their reliability and accuracy.
Deix's experts, with solid academic roots and extensive experience in the fields of mathematical optimization and AI, are developing innovative methodologies to "decrypt" complex AI models. This pioneering work not only opens up new avenues in building transparent AI models but also sets a new standard for the responsible and conscious use of AI in critical contexts such as health, safety, and finance.
Deix's commitment to making artificial intelligence more accessible and understandable goes beyond mere technological improvement. The goal is to build solid trust between users and advanced technologies. By facilitating a greater understanding of how decisions are made by AI systems, Deix not only aims to democratize AI technology but also to strengthen the bond of trust with society. This transparency is essential for true accountability and social acceptance of advanced technologies.
Deix's commitment to "Mathematical Intelligence" is not just a matter of efficiency but an ethical choice aimed at ensuring that the technologies we build improve people's lives sustainably and fairly.
In conclusion, as we continue to navigate the complex world of AI, it is essential that we do not lose sight of the importance of transparency and interpretability. Research efforts in this field not only enhance our technological capabilities but also strengthen the bond of trust between humanity and the machines we choose to build.